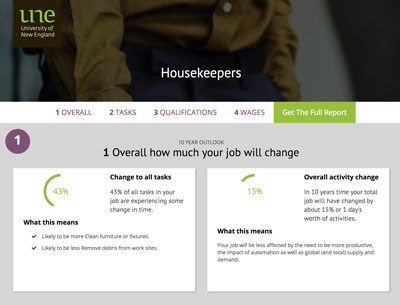
Economic modelling firm AlphaBeta conducted analysis to figure out the degree of change for each occupation in the entire economy.
The Australian economy was broken down into more than 3000 occupations and 1000’s of tasks to determine the degree of likely change in occupations across the entire economy. For example, a factory worker might ‘operate equipment’ and ‘monitor facilities’, while a sales assistant would ‘assist customers’ and ‘assess products’.
Using survey data, the degree in change of time for each task over the last decade was derived to create a metric for overall annual time change. The team then linearly projected forwarded annual time for each task (using appropriate ceilings and floors to create this overall time change metric).
The analysis of job and task change was completed by breaking down the Australian economy into ~3000 occupations and ~2000 tasks.
The primary data source used in this analysis was the O*NET Database (US). The O*NET database contains survey ratings for a range of job-specific tasks for over 1000 occupations. The study used the 2006 and 2014 databases and was then converted to Australian occupations and timeshares. There were 4 main transformations of the data.
The first task was to convert the frequency of performing a task to the amount of time spent on a task.
The O*NET database contains a distribution of survey responses on the frequency of tasks performed e.g. daily, weekly, etc.
A power function was fitted to convert the frequency of task performance to time spent on tasks. The power functional form was selected due to the non-linear inverse relationship between frequency and time spent. Timeshares were then standardised for cross-occupational task variation.
In standardising the results, it was assumed that the total time spent on different tasks equals 40 hours – the weekly full-time equivalent.
Each O*NET Task was mapped to an O*NET Detailed Work Activity (DWA) using the O*NET Database correspondence between tasks and detailed work activities. There are approximately 30 different detailed work activities for each occupation.
Tasks can be assigned to more than one work activity. For tasks that are assigned to more than 1 DWA, an equal proportion of time was allocated to each DWA.
The second task was to define a correspondence between the ‘Standard Occupational Classification’ (SOC) and Australian and New Zealand Standard Classifications of Occupations (ANZSCO).
Each ANZSCO was mapped to the single closest SOC by job title and description. In the case where many SOC Occupations are equivalent to one ANZSCO Occupation, all of them were mapped to one ANZSCO.
The third analytical step was to calculate the change in the time spent on the same task between 2006 to 2014 for each occupation
The change in timeshares was calculated by taking the difference in timeshares between 2006 and 2014. The annual change in time-share was derived by taking a simple average of the change between 2006 and 2014.
Finally, the change in time shares was calculated
Timeshares for each task, in each occupation, was extrapolated linearly using the annual average change in timeshare.
The timeshares were converted into back into weekly hours by multiplying by 40.
To calculate wage growth, employment change and skills levels across regions, the Australian Census 2006, 2011 and 2016 from the Australian Bureau of Statistics (ABS) was used. The data was gathered using the ABS TableBuilder tool. Each metric was validated by comparing the distribution to similar ABS statistics.
Wage Growth
Employment
Skill Level
AlphaBeta is an economics, data and strategy consulting firm based in Sydney, Singapore, Canberra and Melbourne. Through combining expertise in data science, economics, public policy and strategy, they produce unique insights and actionable strategies for public and private sector clients. Their consultants have significant experience in research, public policy, strategy, evaluation and data analysis. They lead Australia in the access to, and use of, data assets relevant to public sector clients. AlphaBeta has a strong background in discovering skill changes and analysing its economic implications.
Other work that AlphaBeta has published on skills and automation includes: